Multi-View Inconsistency Analysis for Video Object-Level Splicing Localization
DOI:
https://doi.org/10.62677/IJETAA.2403111Keywords:
Video splicing forgery, Multi-view feature learning, Object-level forgery detectionAbstract
In the digital era, the widespread use of video content has led to the rapid development of video editing technologies. However, it has also raised concerns about the authenticity and integrity of multimedia content. Video splicing forgery has emerged as a challenging and deceptive technique used to create fake video objects, potentially for malicious purposes such as deception, defamation, and fraud. Therefore, the detection of video splicing forgery has become critically important. Nevertheless, due to the complexity of video data and a lack of relevant datasets, research on video splicing forgery detection remains relatively limited. This paper introduces a novel method for detecting video object splicing forgery, which enhances detection performance by deeply exploring inconsistent features between different source videos. We incorporate various feature types, including edge luminance, texture, and video quality information, and utilize a joint learning approach with Convolutional Neural Network (CNN) and Vision Transformer (ViT) models. Experimental results demonstrate that our method excels in detecting video object splicing forgery, offering promising prospects for further advancements in this field.
Downloads
References
C. Dong, X. Chen, R. Hu, J. Cao, and X. Li, "Mvss-net: Multi-view multi-scale supervised networks for image manipulation detection,"IEEE TPAMI, vol. 45, no. 3, pp. 3539–3553, 2023.
C. Yan, S. Li, and H. Li, "Transu2-net: A hybrid transformer architecture for image splicing forgery detection,"IEEE Access, vol. 11, pp. 33313–33323, 2023.
Y. Liu, X. Zhu, X. Zhao, and Y. Cao, "Adversarial learning for constrained image splicing detection and localization based on atrous convolution,"IEEE Transactions on Information Forensics and Security, vol. 14, no. 10, pp. 2551–2566, 2019.
X. B. andZhipeng Zhang andBin Xiao, "Reality transform adversarial generators for image splicing forgery detection and localization," inICCV, (Montreal, QC, Canada), pp. 14274–14283, 2021.
X. Shi, P. Li, H. Wu, Q. Chen, and H. Zhu, "A lightweight image splicing tampering localization method based on mobilenetv2 and SRM,"IET Image Process., vol. 17, no. 6, pp. 1883–1892, 2023.
M. K. Johnson and H. Farid, "Exposing digital forgeries by detecting inconsistencies in lighting," inProceedings of the 7th workshop on Multimedia & Security, MM&Sec 2005, New York, NY, USA, August 1-2, 2005, 2006 (A. M. Eskicioglu, J. J. Fridrich, and J. Dittmann, eds.), pp. 1–10, ACM, 2005.
Y. Niu, B. Tondi, Y. Zhao, R. Ni, and M. Barni, "Image splicing detection, localization and attribution via JPEG primary quantization matrix estimation and clustering,"IEEE Trans. Inf. Forensics Secur., vol. 16, pp. 5397–5412, 2021.
Y. Zhang, G. Zhu, L. Wu, S. Kwong, H. Zhang, and Y. Zhou, "Multi-task se-network for image splicing localization,"IEEE Trans. Circuits Syst. Video Technol., vol. 32, no. 7, pp. 4828–4840, 2022.
Q. Diao, Y. Jiang, B. Wen, J. Sun, and Z. Yuan, "Metaformer: A unified meta framework for fine-grained recognition," inCVPR, (New Orleans, Louisiana, USA),IEEE, 2022.
K. Sun, B. Xiao, D. Liu, and J. Wang, "Deep highresolution representation learning for human pose estimation," inCVPR, (Long Beach, CA, USA), pp. 56935703, IEEE, 2019.
Y. Yuan, R. Fu, L. Huang, W. Lin, C. Zhang, X. Chen, and J. Wang, "Hrformer: High-resolution vision transformer for dense predict," inNeurIPS, (Virtual), pp. 7281–7293, 2021.
H. Ding, L. Chen, Q. Tao, Z. Fu, L. Dong, and X. Cui, "Dcu-net: a dual-channel u-shaped network for image splicing forgery detection,"Neural Comput. Appl.,vol. 35, no. 7, pp. 5015–5031, 2023.
B. C. Hadwiger and C. Riess, "Deep metric color embeddings for splicing localization in severely degraded images,"IEEE Transactions on Information Forensics and Security, vol. 17, pp. 2614–2627, 2022.
B. C. Hadwiger and C. Riess, "Deep metric color embeddings for splicing localization in severely degraded images,"IEEE Transactions on Information Forensics and Security, vol. 17, pp. 2614–2627, 2022.
Y. Rao and J. Ni, "Self-supervised domain adaptation for forgery localization of JPEG compressed images," inICCV, (Montreal, QC, Canada), pp. 15014–15023, 2021.
P. Pei, X. Zhao, J. Li, Y. Cao, and X. Lai, "Vision transformer based video hashing retrieval for tracing the source of fake videos,"Security and Communication Networks, vol. 2023, 2023.
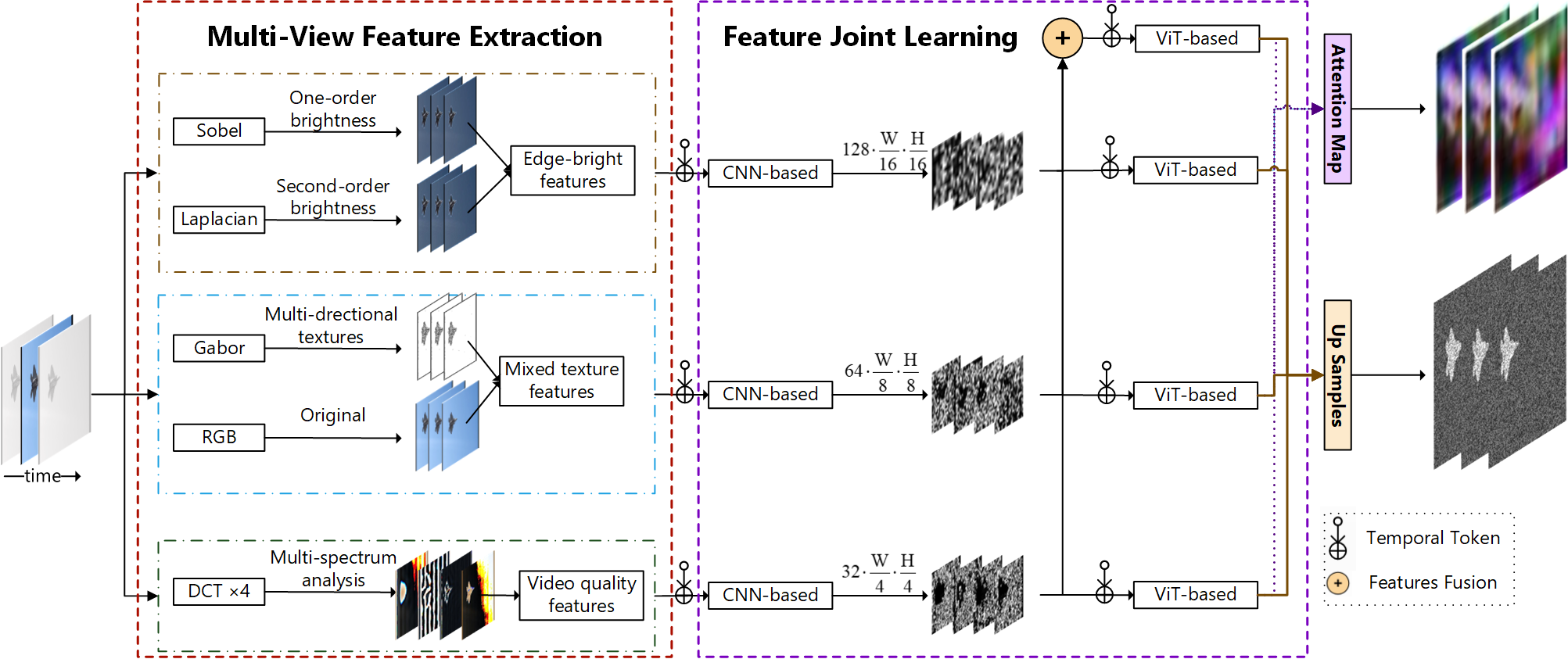
Downloads
Published
Versions
- 2024-05-13 (2)
- 2024-04-24 (1)
Issue
Section
Categories
License
Copyright (c) 2024 Pengfei Peng, Guoqing Liang, Tao Luan (Author)

This work is licensed under a Creative Commons Attribution-NonCommercial-NoDerivatives 4.0 International License.