A Comprehensive Review of Simulation Technology: Development, Methods, Applications, Challenges and Future Trends
DOI:
https://doi.org/10.62677/IJETAA.2405119Keywords:
Simulation technology, Modeling and simulation, Artificial intelligence, Digital twin, Hybrid modelingAbstract
Simulation technology is a comprehensive method for predicting possible outcomes or evaluating decision-making effects by establishing computer models of complex systems and simulating their behavior and evolution processes. This paper systematically reviews the development history, main methods, application fields, challenges, and future trends of simulation technology. Firstly, the article defines the connotation of simulation technology and expounds its important value in military, business, public policy, and other fields. Secondly, the article traces the development of simulation technology from its origins in World War II to the present, summarizing key milestones such as modeling languages, graphical interfaces, distributed interaction, and cloud computing. Thirdly, the article introduces the main modeling paradigms, such as system dynamics, discrete events, and agents, as well as compound modeling methods like continuous-discrete hybrid and multi-agent-macro hybrid. Meanwhile, the article also demonstrates typical applications of simulation in defense, business, government, engineering, healthcare, and other fields, using case studies in military operations, consumer markets, macroeconomics, traffic management, and disease spread. Based on an analysis of existing technical challenges, practical dilemmas, and methodological controversies, the article finally outlines the development prospects of simulation technology in the directions of large-scale high-performance computing, data-driven modeling, human-computer hybrid, cognitive-behavioral simulation, and cross-domain coupling. The article points out that simulation is accelerating its integration with artificial intelligence, big data, digital twins, and other technologies, evolving towards more intelligent, real-time, and immersive directions, and will become an indispensable enabling technology in the digital era. Strengthening theoretical innovation and application expansion of simulation is of great significance for enhancing national scientific and technological strength and comprehensive competitiveness.
Downloads
References
R. Fujimoto, Parallel and distributed simulation, Proceedings of the 2015 Winter Simulation Conference, pp. 45-59, 2015.
B. P. Zeigler, A. Muzy, and E. Kofman, Theory of modeling and simulation: discrete event & iterative system computational foundations,Academic press, 2018.
L. Yilmaz and T. ̈Oren, Agent-directed simulation and systems engineering, John Wiley & Sons, 2020.
J. Banks, J. S. Carson, B. L. Nelson, et al., Discrete-event system simulation, Pearson, 2022.
A. Anagnostou and S. J. E. Taylor, “A distributed simulation methodological framework for OR/MS applications,” Simulation Modelling Practice and Theory, vol. 70, pp. 101-119, 2017.
J. A. Sokolowski, C. M. Banks, and R. L. Hayes, “Modeling and simulation support for system of systems engineering applications,” Simulation Modelling Practice and Theory, vol. 105, pp. 102189, 2021.
A. Tolk, N. Mustafee, Y. Uygun, et al., “Hybrid simulation: historical lessons, present challenges and futures,” in 2021 Winter Simulation Conference (WSC), IEEE, 2021, pp. 1-15.
T. Eldabi, M. Balaban, S. Brailsford, et al., “Hybrid simulation: historical lessons, present challenges and futures,” in 2016 Winter Simulation Conference (WSC), IEEE, 2016, pp. 1388-1403.
K. Ross, K. M. Hopkinson, and M. Pachter, “Using a distributed agentbased communication enabled special protection system to enhance smart grid security,” IEEE Transactions on Smart Grid, vol. 4, no. 2,pp. 1216-1224, 2013.
S. Liu, C. Mao, Q. Gao, et al., “An integrated DDMSM-based modeling and simulation approach for supporting analysis of complex systems,” Simulation Modelling Practice and Theory, vol. 99, pp. 102033, 2020.
H. Lin, Y. Jiang, X. Zhao, et al., “Hybrid modeling and simulation with improved differential evolution optimization algorithm for system effectiveness evaluation,” IEEE Access, vol. 9, pp. 8629-8645, 2021.
A. G. Bruzzone, M. Massei, F. Longo, et al., “Simulation and digital twins as enablers for agile production and supply chain optimization,” Sustainability, vol. 14, no. 7, pp. 4181, 2022.
Y. Suh and H. Lee, “Data-driven modeling and simulation for performance evaluation of public transportation systems,” IEEE Access, vol.9, pp. 148462-148473, 2021.
A. Anagnostou, A. Nouman, and S. J. E. Taylor, “Distributed hybrid agent-based discrete event emergency medical services simulation,” in 2013 Winter Simulations Conference (WSC), IEEE, 2013, pp. 1625-1636.
IEEE Standard for Modeling and Simulation (M&S) High Level Architecture (HLA)–Framework and Rules, IEEE Std 1516-2000, pp. i-22,2000.
D. Silver, A. Huang, C. J. Maddison, et al., “Mastering the game of Go with deep neural networks and tree search,” Nature, vol. 529, no. 7587, pp. 484-489, 2016.
M. Suleiman, V. Gaudreault, J. Bouchard, et al., “15 Years of the Journal of Artificial Societies and Social Simulation (JASSS),” Journal of Artificial Societies and Social Simulation, vol. 21, no. 4, 2018.
A. Borshchev, Y. Karpov, and V. Kharitonov, “Distributed simulation of hybrid systems with AnyLogic and HLA,” Future Generation Computer Systems, vol. 18, no. 6, pp. 829-839, 2002.
B. P. Zeigler, H. S. Sarjoughian, R. Duboz, et al., Guide to modeling and simulation of systems of systems, Springer London, 2012.
R. Fujimoto, C. Bock, W. Chen, et al., “Research challenges in modeling and simulation for engineering complex systems,” Journal of simulation,vol. 93, no. 9, pp. 717-722, 2017.
F. Tao, M. Zhang, Y. Liu, et al., “Digital twin in industry: state-of-the-art,” IEEE Transactions on Industrial Informatics, vol. 15, no. 4, pp.2405-2415, 2018.
D. Cearley and B. Burke, “Top 10 strategic technology trends for 2018,” Gartner research, vol. 3, pp. 4-11, 2017.
A. Balaji, M. Farajtabar, D. Yin, et al., “Massively parallel hyperparameter tuning,” Proceedings of the Machine Learning and Systems 4, 2022.
IEEE Standard for Lifecycle Process for Digital Twins in Manufacturing, IEEE Std 2806-2022, pp. 1-23, 2022.
C. S. M. Currie, J. W. Fowler, K. Kotiadis, et al., “How simulation modelling can help reduce the impact of COVID-19,” Journal of Simulation, vol. 14, no. 2, pp. 83-97, 2020.
M. N. Parker, V. C. Shankar, P. B. Bobko, et al., The Actionable Simulation Guidance System (ASGS) Software Tool to Assure Modeling and Simulation (M&S) Credibility for Influence Diagrams (IDs), MITRE Corp Bedford United States, 2021.
H. Lin, Q. Sun, J. Li, et al., “The digital twin of a city: Cityverse, city information modeling and social functions,” Building and Environment,pp. 110434, 2023.
X. Xu, Y. Wang, Y. Liu, et al., “A review of metaverse: Fundamentals, security, and privacy,” arXiv preprint arXiv:2203.02662, 2022.
K. Nagel, W. Kruse, M. Schreckenberg, S. Seshadri, and I. J. Timm, “Open source agent-based and activity-based urban mobility simulation,” Simulation Modelling Practice and Theory, vol. 118, pp. 102532, 2022.
R. Conte and M. Paolucci, “On agent-based modeling and computational social science,” Frontiers in psychology, pp. 668, 2014.
H. Dawid, S. Gemkow, P. Harting, et al., “Agent-based macroeconomic modeling and policy analysis: the Eurace@ Unibi model,” in Handbook on computational economics and finance. Oxford University Press, 2018.
R. Ren, H. Guo, Z. Zhang, et al., “Digital twin-driven product design framework,” International Journal of Production Research, vol. 59, no.10, pp. 3101-3116, 2021.
X. Pan, Y. You, Z. Wang, et al., “Virtual to real reinforcement learning for autonomous driving,” in British Machine Vision Conference, 2017.
H. Kavak, J. J. Padilla, C. J. Lynch, et al., “Big data, agents, and machine learning: towards a data-driven agent-based modeling approach,” in Proceedings of the Annual Simulation Symposium, 2018, pp. 1-12.
J. M. Epstein, Agent Zero: Toward neurocognitive foundations for generative social science, Princeton University Press, 2014.
A. Angourakis, J. Bates, D. Baudry, et al., “How to co-simulate complex models? Exploring techniques for coupled agent-based and dynamic microsimulation models in archaeology,” Simulation Modelling Practice and Theory, vol. 117, pp. 102507, 2022.
A. Rasheed, O. San, and T. Kvamsdal, “Digital twin: Values, challenges and enablers from a modeling perspective,” IEEE Access, vol. 8, pp.21980-22012, 2020.
T. Choi, W. K. Yeung, and V. Tang, “Digital twin modeling for smart manufacturing,” Procedia Manufacturing, vol. 57, pp. 2859-2866, 2021.
J. Derbyshire and G. Wright, “Augmenting the intuitive logics scenario planning method for a more comprehensive analysis of causation,” International Journal of Forecasting, vol. 33, no. 1, pp. 254-266, 2017.
T. Eldabi, S. Brailsford, A. Djanatliev, et al., “Hybrid simulation challenges and opportunities: a life-cycle approach,” in 2018 Winter Simulation Conference (WSC), IEEE, 2018, pp. 1500-1514.
Z. Sun, Y. Feng, N. Lu, et al., Reflections on simulation methodology: beyond the dichotomy between Borrowing from other disciplines” and “Developing distinct methodology”,” Systems Engineering-Theory & Practice, vol. 34, no. 6, pp. 1407-1417, 2014.
T. Gr ̈une-Yanoff and P. Weirich, “The philosophy and epistemology of simulation: a review,” Simulation & Gaming, vol. 41, no. 1, pp. 20-50, 2010.
W. G. Kennedy, “Computational social science and generative explanations of emergent behavior using agent modeling,” in 2022 Spring Simulation Conference (SpringSim), IEEE, 2022, pp. 1-10.
J. Weidinger, S. Schlauderer, and S. Overhage, “Is the frontier shifting into the right direction? A qualitative analysis of acceptance factors for novel firefighter information technologies,” Information Systems Frontiers, vol. 20, no. 4, pp. 669-692, 2018.
F. Tao, F. Sui, A. Liu, et al., “Digital twin-driven product design framework,” International Journal of Production Research, vol. 57, no.12, pp. 3935-3953, 2019.
R. S. Miller, “Embracing the future of modeling and simulation: computational challenges and opportunities on the horizon,” in Proceedings of the 2018 ACM SIGSIM Conference on Principles of Advanced Discrete Simulation, 2018, pp. 151-152.
S. Liu, G. Zhang, G. Tian, et al., “A military simulation training system based on VR/AR technology,” in 2021 IEEE International Conference on Artificial Intelligence and Computer Applications (ICAICA), IEEE,2021, pp. 589-594.
J. P. Bouchaud, S. Gualdi, M. Tarzia, et al., “Optimal inflation target:insights from an agent-based model,” Economics: The Open-Access,Open-Assessment E-Journal, vol. 14, no. 2020-15, pp. 1-27, 2020.
S. J. Alam and A. Geller, “Networks in agent-based social simulation,” in Agent-based models of geographical systems. Springer, Dordrecht,2012, pp. 199-216.
A. Nouman, A. Anagnostou, and S. J. E. Taylor, “Developing a distributed agent-based and DES simulation using poRTIco and repast,” in 2013 IEEE/ACM 17th International Symposium on Distributed Simulation and Real Time Applications, IEEE, 2013, pp. 97-104.
H. Kavak, J. J. Padilla, C. J. Lynch, et al., “The past, present, and future of simulation-based training in emergency management,” Simulation Modelling Practice and Theory, vol. 108, pp. 102272, 2021.
M. Farsi, A. Daneshkhah, A. Hosseinian-Far, et al., Digital twin technologies and smart cities, Springer, 2020.
W. G. Kennedy and F. E. Ritter, “Developing a cognitive modeling consideration set,” MethodsX, vol. 8, pp. 101477, 2021.
F. Dechesne, C. Sch ̈utz, and L. Dierks, “Hybrid modelling in sustainability assessment,” in Advanced Hybrid Simulation. Springer, Cham, 2022, pp. 147-165.
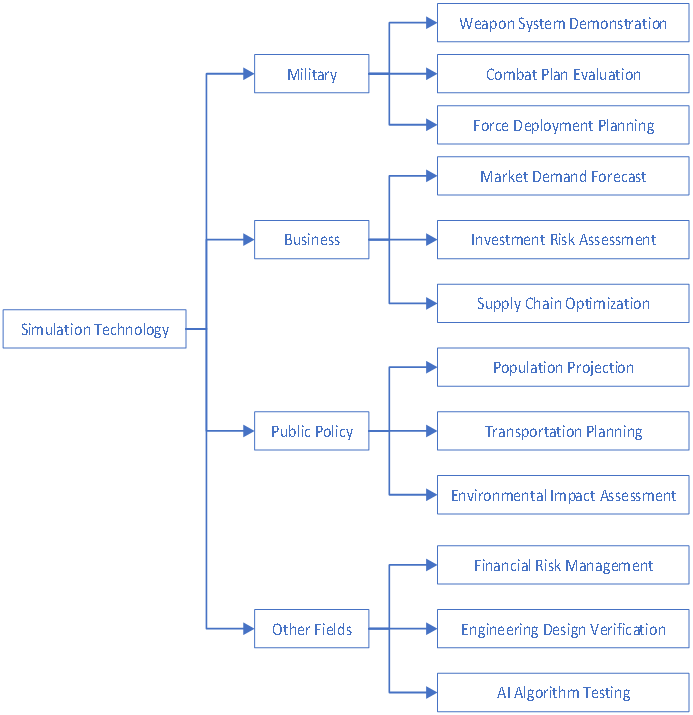
Downloads
Published
Issue
Section
Categories
License
Copyright (c) 2024 Tao Luan (Author)

This work is licensed under a Creative Commons Attribution-NonCommercial-NoDerivatives 4.0 International License.